Boosting scientific discovery through intelligent experimental design
Roman Garnett will build new algorithms for active machine learning that will accelerate extracting knowledge from big data with an NSF CAREER Award
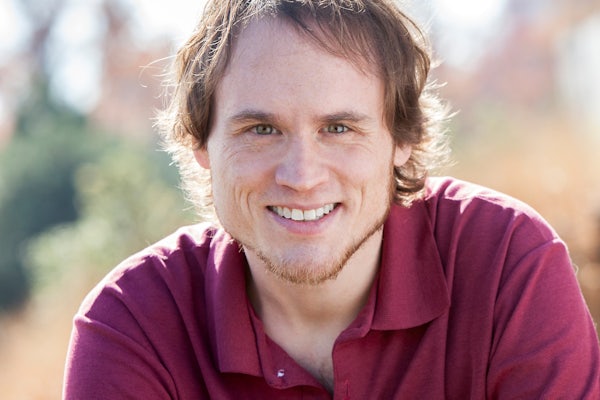
Garnett earns NSF CAREER Award
Within two decades, tools from machine learning will likely be a standard tool in engineering and scientific discovery. Scientists are building better and better instruments to collect more and more data. But someone — or something — must analyze that data to extract scientific knowledge.
Roman Garnett, in the McKelvey School of Engineering at Washington University in St. Louis, will build new algorithms for a method known as active machine learning that will accelerate extracting knowledge from big data with a five-year, $497,693 CAREER Award from the National Science Foundation (NSF). The awards support junior faculty who model the role of teacher-scholar through outstanding research, excellent education and the integration of education and research within the context of the mission of their organization. One-third of current McKelvey Engineering faculty have received the award.
In some situations, analyzing new data requires expensive processes such as running a simulation on a supercomputer or having a human perform the analysis in a lab. With active machine learning, scientists can automatically and adaptively design experiments to make the best use of limited resources. Active machine learning can be considered an automated approach to the scientific method.
"We take data that we've already collected and use it to give us an idea about what is happening," said Garnett, assistant professor of computer science & engineering. "Then we build a model to reason about what outcomes of new experiments might be based on what we've already learned. We use that model with our goals to develop a rule or method to look at a large collection of data and identify what is the most useful. The hope is that we are able to achieve our goal more efficiently than we would have with, for example, randomly-selected data."
For instance, Garnett said astronomers are building better telescopes to get a better look at stars, galaxies and quasars in the sky. Thousands of these objects can be imaged in one night, creating hundreds of gigabytes of data. That's where Garnett's work comes in.
"Having the data is not the point," he said. "You want to extract some knowledge. Now we've got a thousand times more data than we used to have, so we have an even bigger challenge. Astronomers are now resorting to 'citizen-scientist' volunteers to analyze some of these images to classify the objects and search for rare phenomena. There is a big challenge in effectively prioritizing the data for these volunteers to make the best use of their time."
Garnett's research will develop automated tools to quickly extract scientific knowledge in situations such as these.
Garnett and his team also will continue to develop the field of active search, which he founded, into a new tool to automatically find new members of a valuable class within a dataset, such as finding new materials for drug discovery.
"It's a blessing and a curse because you know that whatever you're looking for is going to be in the data somewhere, but now you have to find it," he said.
"For example, you might take an image of a thousand lights in the sky, but maybe you're searching for one special kind of object. It could be that you don't care about 99 percent of the data because you're searching for examples of this rare phenomenon. Now your goal is to try to find a needle in the haystack."
In addition, Garnett's team will increase access to active machine learning by building fully automated procedures. His lab will further the field of automated machine learning (AutoML), which uses machine learning to automate the process of machine learning itself, Garnett said. This will be particularly useful for scientists and engineers who want to adopt active machine learning to help analyze their data.
"AutoML helps them adaptively improve the models that we're using for the experimental design. We can automatically build useful models just from their data, opening the power of these techniques to a wide audience" he said.
As part of his research, Garnett will develop an undergraduate course on sequential decision making as well as work with other faculty members to incorporate active machine learning into other engineering and science courses. He also plans to work with citizen-scientists who are working with astronomers to find new galaxies through a collaboration with Zooniverse. In addition, he is co-writing a book on Bayesian optimization.