Physics, AI help researchers to better define tumor boundaries in imaging
A team of researchers has developed a framework to more precisely determine tumor boundaries in PET scans using physics and artificial intelligence (AI)
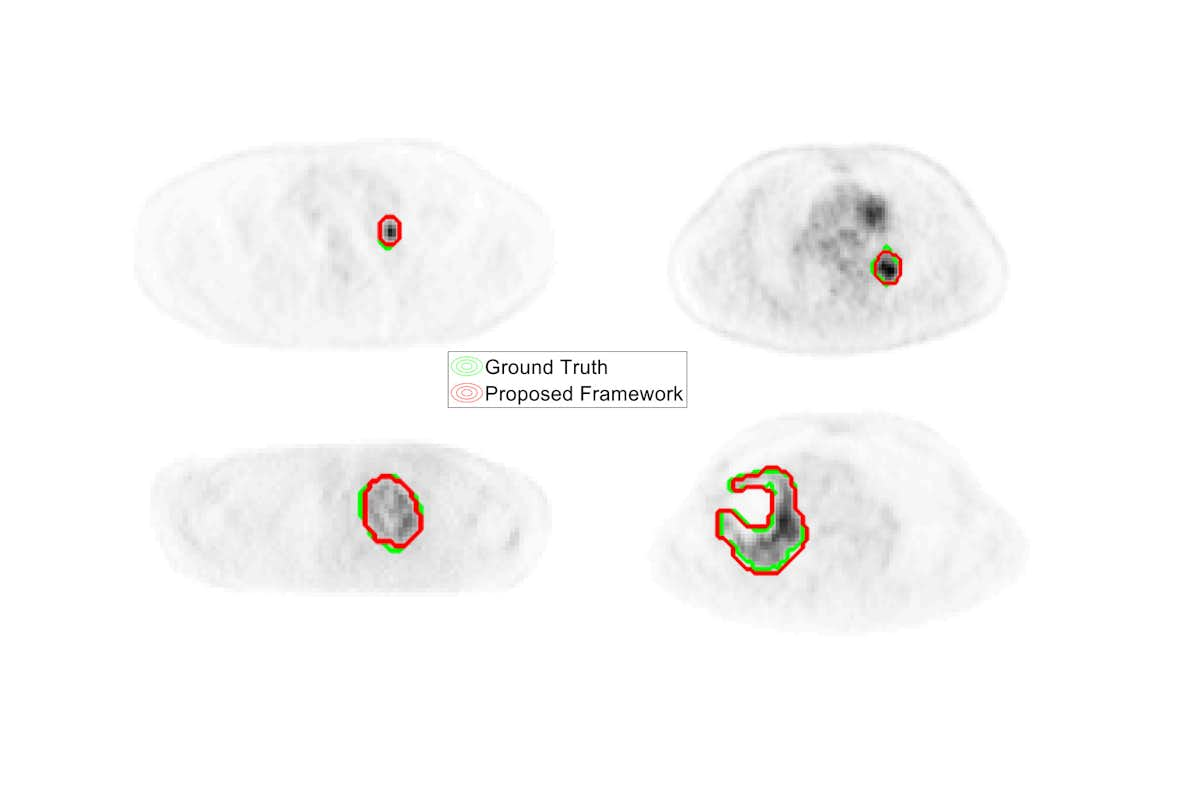
In several procedures to treat cancerous tumors, clinicians must know the boundaries of the tumor. Positron emission tomography (PET) provides an approach to image tumors in vivo, but current method to define tumor boundaries from PET scans have limitations. A team of researchers at several institutions worldwide, including Washington University in St. Louis, has developed a framework to more precisely determine tumor boundaries in PET scans using physics and artificial intelligence (AI).
Abhinav Jha, assistant professor of biomedical engineering McKelvey School of Engineering at WashU, and collaborators at Johns Hopkins University, Jordan University of Science and Technology, Memorial Sloan Kettering Cancer Center and the University of British Columbia combined their expertise in physics, imaging and machine learning to improve delineation of lung tumors in PET scans. Results are published online in Physics in Medicine & Biology.
"In the clinic, there is a need for methods to delineate the tumor boundary for PET-based radiotherapy planning," said Jha, who also is an assistant professor of radiology at Washington University School of Medicine. "Tumor delineation is also needed, and in radiomics where we extract features from the image and use these features to see if the patient has a better chance of responding to treatment or has a better chance of surviving."
PET imaging observes the metabolic processes in the body to detect disease at an earlier stage. However, these images are typically noisy, or grainy, and are low resolution, making the boundaries of tumors blurry. While AI has shown promise in delineating high-resolution and less-noisy images, PET images pose challenges due to the lower resolution, noise, and lack of availability of ground-truth tumor boundaries. That's where integrating physics with AI comes in, Jha said.
In this project, initiated at Johns Hopkins University, Jha and the team used a physics-based approach to generate thousands of clinically realistic simulated PET scans with known ground-truth boundaries used to train a convolutional neural network to delineate accurate boundaries of the tumor. Finally, the network is fine-tuned with a small number of clinical PET scans.
They validated this approach using clinical data from a group of patients with lung cancer.
"Our method is more accurate and able to delineate tumors as small as 1.7 centimeters square," Jha said. "Further, the method required a small number of clinical images for training. We were able to test if our method is able to generalize across different scanners, and we found that it does.
"This research demonstrates how combining expertise of researchers from multiple disciplines such as physics, radiology and AI can help address challenging problems in medicine," Jha said.
Jha presented part of this work at the FDA Public Workshop on Evolving Role of AI in Medical Imaging in February 2020, as well as at the Medical Imaging Technology showcase in Washington, DC, after being selected to the Council for Early Career Investigators in Imaging of the Academy of Radiology and Biomedical Imaging.
The McKelvey School of Engineering at Washington University in St. Louis promotes independent inquiry and education with an emphasis on scientific excellence, innovation and collaboration without boundaries. McKelvey Engineering has top-ranked research and graduate programs across departments, particularly in biomedical engineering, environmental engineering and computing, and has one of the most selective undergraduate programs in the country. With 140 full-time faculty, 1,387 undergraduate students, 1,448 graduate students and 21,000 living alumni, we are working to solve some of society’s greatest challenges; to prepare students to become leaders and innovate throughout their careers; and to be a catalyst of economic development for the St. Louis region and beyond.
Funding was provided, in part, by the National Institutes of Health (P41-EB024495, R21-EB024647).
Leung KH, Marashdeh W, Wray R, Ashrafinia S, Pomper MG, Rahmim A, Jha AK. A physics-guided modular deep-learnings based automated framework for tumor segmentation in PET. 2020. Physics in Medicine & Biology, in press. https://doi.org/10.1088/1361-6560/ab8535.
Source code for this work available at https://jhalab.wustl.edu/software/a-fully-automated-modular-framework-for-pet-segmentation/