Deep learning investigated to improve imaging techniques
Ulugbek Kamilov will investigate a new framework with an NSF CAREER Award
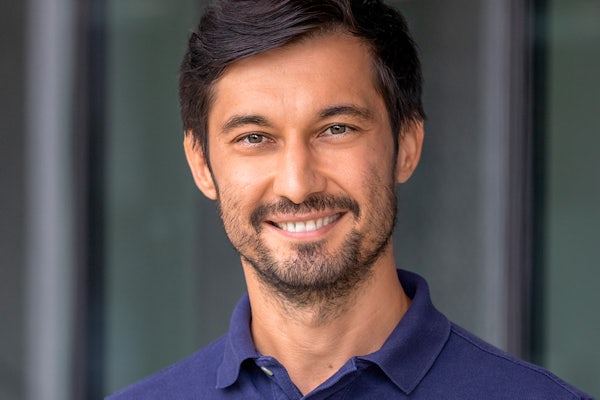
When getting an MRI scan, a patient is told to lie as still as possible because any movement will create errors in the scans. An engineer at Washington University in St. Louis is proposing a novel way to correct errors in MRIs and other types of images using deep learning.
Ulugbek Kamilov, assistant professor of computer science & engineering and of electrical & systems engineering in the McKelvey School of Engineering, plans to develop a framework that ultimately would lead to algorithms to correct these errors and enhance imaging capabilities with a five-year, $486,000 CAREER Award from the National Science Foundation. CAREER awards support junior faculty who model the role of teacher-scholar through outstanding research, excellence in education and the integration of education and research within the context of the mission of their organization. One-third of current McKelvey Engineering faculty have received the award.
Kamilov, whose research is in computational imaging, said the demand for more advanced imaging technology is increasing, leading to the need to remove artifacts, noise or other errors, which cannot be done by hand. That’s where deep learning, which simulates human brain functionality by using a neural network, comes in.
“In MRI scans, you scan patients then feed their images to a neural network to learn the patterns we want to eliminate,” he said. “We then use those trained neural networks to fix the errors in subsequent scans.”
Currently, if there are errors in data, the data is discarded, Kamilov said.
“We want to know what we are losing and if we can guarantee that the images we get are what we want to get,” he said. “Deep learning is a powerful technology, however, it is not sufficient for this since it doesn’t exploit what we already know about the physics of the instrument. This work is trying to bring together deep learning and known physical information to give us more interpretable results and better performance.”
In preliminary work, Kamilov, his lab and collaborators have seen MRI processing speeds three to five times faster and with the same quality images as existing technology.
“Having good quality images in less time has the benefit of reducing costs because you can scan people faster and reduce insurance costs and employee time per patient,” he said.
As part of this work, Kamilov has several collaborations to integrate the framework for improvements to existing imaging technology. One collaboration with Hongyu An, associate professor in the Mallinckrodt Institute of Radiology at the School of Medicine, is looking at a way to improve a five-minute MRI scan that produces multiple 3D images, each at a different position of the breathing cycle. By using deep learning and the new algorithm, the MRI time can be reduced to one to two minutes. This technique has been incorporated in a pre-product by Siemens and has been tested by other customers.
Another collaboration with Dmitriy Yablonskiy, professor of radiology at the School of Medicine, is interested in repairing the data from MRI scans during which patients have moved. Yablonskiy’s lab uses imaging techniques that link brain genetic and cellular microstructure with brain functioning. One of these techniques can identify Alzheimer’s disease-related tissue cellular damage before onset of the tissue atrophy measured by MRI.
Kamilov has another collaboration with Brendt Wohlberg at Los Alamos National Laboratory that focuses on developing computationally efficient algorithms for the analysis of large datasets from scientific experiments. The analysis of many of these datasets requires supercomputer facilities, making it difficult for experimentalists to detect calibration issues and other problems while conducting their experiments. These new algorithms have the potential to be run on much smaller computers and to process experimental data as it is received, allowing experimentalists to detect problems much earlier than currently possible.
He also plans to work with scientists at the Donald Danforth Plant Science Center to accelerate the now-slow processing time of microCT scanners used to study the growth of roots. Finally, he plans to work with James Fitzpatrick, professor of neuroscience at the School of Medicine, to apply the algorithm to cryogenic electron microscopy (cryo-EM), which allows scientists to see atomic structures to understand the 3D shape of molecules.
“From my perspective, I am describing different instruments, and the only thing I change is the way each maps the data,” Kamilov said. “For each one, I learn the constraints on the unknown image by giving examples of prior images, by swapping them or putting them in different applications.”
For the outreach component of the CAREER Award, Kamilov is planning a summer imaging workshop for high school students with a high school teacher working as an intern in the lab. In addition, he plans to further develop a course, Large Scale Optimization for Data Science, that teaches how to write algorithms when there are billions of unknowns.