McKelvey Engineering faculty lead MURI to study brain dynamics, reinforcement learning
It is the first MURI directly awarded to an investigator at the McKelvey School of Engineering in a decade
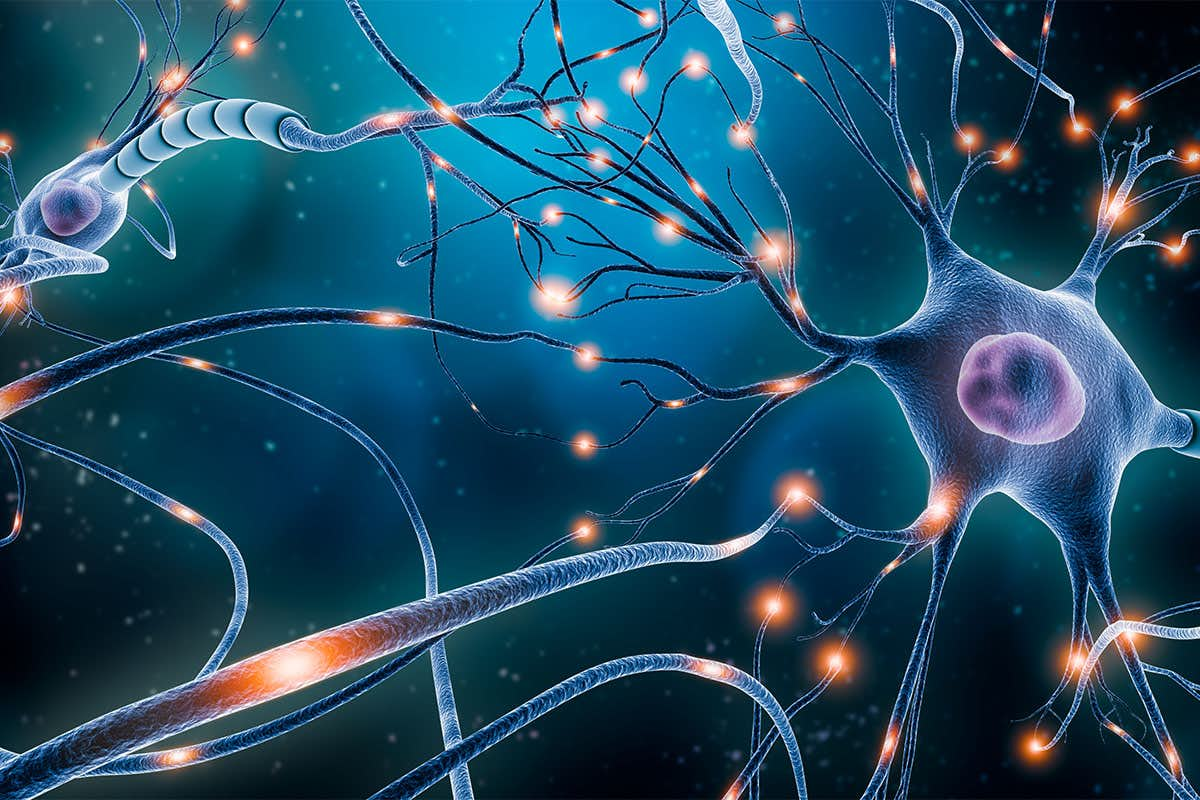
Over the past half century, artificial intelligence has gone from a concept to everyday life, with electronic payments, facial recognition and social media becoming the norm. But the dynamic mechanisms in the brain on which artificial intelligence is based, which are more efficient, reliable and flexible, remain unclear to scientists, leading to a disconnect between biological and machine learning.
A multidisciplinary and multi-institutional team of researchers will probe these brain cell dynamics with a three-year, $3.75 million Multidisciplinary University Research Initiative (MURI) grant from the U.S. Army Combat Capabilities Development Command’s Army Research Laboratory. The highly competitive MURI awards support research teams whose efforts intersect more than one traditional science and engineering discipline. It is the first MURI in which McKelvey School of Engineering at Washington University in St. Louis is the lead institution in about 10 years.
ShiNung Ching, associate professor of electrical & systems engineering in the McKelvey School of Engineering, will lead the effort in collaboration with Bruno Sinopoli, the Das Family Distinguished Professor and chair of the Preston M. Green Department of Electrical & Systems Engineering and an expert on robust and resilient design of cyber-physical systems; Ilya Monosov, associate professor of neuroscience, whose lab studies the neuronal mechanisms that control exploration and learning, and Thomas Papouin, assistant professor of neuroscience, whose lab studies the role of glial cells in brain function, both at Washington University School of Medicine; Fabio Pasqualetti, associate professor of mechanical engineering, whose lab studies the design of network-theoretic theories and tools for the study of brain processes from imaging data, and Samet Oymak, assistant professor of electrical and computer engineering whose lab studies the confluence of machine learning, optimization and statistics, both at the University of California, Riverside.
In this work, the team seeks to understand how neural circuits in the brain acquire and perform complex functions by accruing experience over long swatches of time through trial and error, a framework known as reinforcement learning. While this is a frequently-used paradigm in machine learning and artificial intelligence, researchers do not fully understand how humans and animals are able to quickly learn and make decisions based on many past experiences. Such capability is in contrast with machine learning, which is much slower and often yields decisions based only on immediate circumstances, known as Markovian decision-making. For example, humans can use a lifetime of past experiences and nuanced contexts when driving a car, while driverless cars may use only a limited library of scenarios from which to determine immediate actions.
“We plan to study how this form of history-dependent learning and decision-making may be occurring in brain networks,” Ching said.
Ching, whose research combines engineering and computational neuroscience, said scientists have focused attention on the functional role of neurons in the brain and how they enable actions of humans and animals. However, neurons merely account for half of the brain, sometimes as little as 10% to 20% in some brain areas. The MURI project will directly test the possible importance of glial cells, the non-neuronal cells of the brain that are typically overlooked in attempts to explain brain computational capabilities, in enabling robust and flexible reinforcement learning and decision-making in neural networks.
In their project, the research team will look at the interactions between neurons and astrocytes, a type of glial cell, to see if they form two levels of a network that enables large-scale learning. Combining engineering, machine learning, neurophysiology, behavioral testing and genetics, the team will develop a novel framework that links these neuro-glial dynamics to high-level learning functions through computational modeling and analysis approaches. They will then examine and clarify these dynamics in animal models.
“We want to then translate that knowledge about astrocyte-neuron interactions into algorithmic solutions in the form of new methods for machine learning and optimization,” Ching said.
“We are really excited about the possibility of implementing and testing a more realistic framework for neural networks that is inspired by the true cellular diversity of the brain,” said Papouin, whose lab studies the biology of astrocytes and their role in cognitive behavior. “It’s an important and unique opportunity to challenge the fragile assumption that neurons are the only almighty computing entity of the brain.”
Ching said the team is tackling a problem that has not seen a lot of attention in computational engineering neuroscience.
“This gives us an opportunity to do something quite groundbreaking,” he said.