Wearable fitness trackers help physicians track patient health
Chenyang Lu applies machine learning, Internet of Things expertise to improve patient outcomes
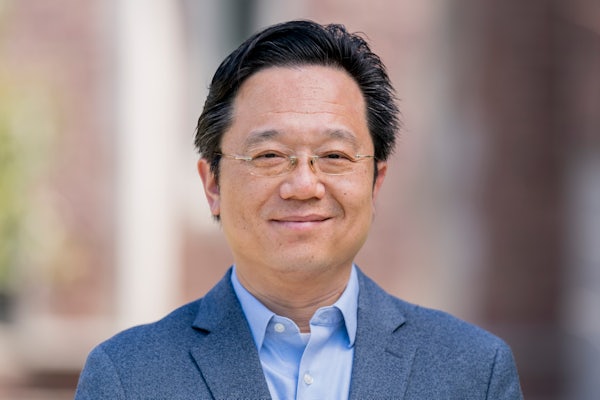
Wearable activity trackers have become common accessories for people of all ages. Over the past few years, researchers at Washington University in St. Louis have been engaged in research to evaluate whether such devices — if worn in the weeks before and after surgery — can help to predict treatment outcomes.
Chenyang Lu, the Fullgraf Professor in the McKelvey School of Engineering, has been part of several teams with surgeons and physicians at the School of Medicine that have been testing Fitbit activity trackers in studies that have shown that these relatively inexpensive wearable devices can play a valuable role in improving patient health.
“My lab has developed machine learning models and tools to predict clinical outcomes using Fitbit wristbands in multiple collaborative research projects with clinicians,” Lu said. “We can collect multi-modal data, such as step count, heart rate and sleep cycles, which we use with our machine learning models to predict deterioration or improvement in a patient’s health status. These efforts demonstrate tremendous potential for wearable and machine learning to improve health care.”
Lu’s success in this area, known as the Internet of Medical Things (IoMT), has attracted the attention of clinicians at the School of Medicine who are interested in evaluating the potential benefits of this technology.
“From an engineering standpoint, the most interesting research question is how do you extract clinically relevant information and make robust predictions based on these fine-grained and noisy data collected with consumer-grade wearables,” Lu asks. “We want to make broad impacts on health care by leveraging the same kind of technology that we build and applying it to a multitude of different medical problems.”
For example, physicians have faced significant challenges to get long-term, nonintrusive, continuous monitoring of patients once they leave the hospital, Lu said.
“The ability to get continuous information about their patients outside of the hospital gives them unprecedented opportunities to improve health care,” Lu said. “Wearable devices, wireless connectivity, cloud computing and machine learning are the perfect combination of technology that makes this possible.”
Philip Payne, the Janet and Bernard Becker Professor and director of the Institute for Informatics, associate dean for health information and data science and chief data scientist at the School of Medicine, said there is no good way to integrate the data that comes from patients’ wearable devices with their electronic health record at this time. However, Lu is one of the few people at WashU and in the U.S. who is making headway in this area.
“Chenyang has established himself as an expert in how to interpret and connect the dots for this high-dimensional data,” Payne said. “That’s why I think he’s so prolific. He is also very knowledgeable around the use of machine-learning methods as applied to messy real-world data sets – the kinds we get when people live their lives. We are learning how to interpret this messy data and apply machine learning and artificial intelligence to predict outcomes, and Chenyang is engaged in teams looking at those types of problems.”
Most recently, Lu worked with Chet Hammill, MD, associate professor of surgery at the School of Medicine and a surgeon at Barnes-Jewish Hospital, and several other colleagues on a study funded by the university’s Big Ideas Competition and the Foundation for Barnes-Jewish Hospital to evaluate whether Fitbit devices worn by pancreatic surgery patients could help to predict their surgical outcomes. For the study, published in the Journal of Medical Internet Research in 2021, the team provided Fitbits to 48 patients with pancreatic cancer about a month before they were scheduled to have pancreas resection surgery at Barnes-Jewish Hospital. The Fitbit devices tracked the number of steps each patient took per day, their sleep patterns and heart rate. Lu and the team took that data, along with patient characteristics, and developed a robust machine learning pipeline to predict surgical outcomes.
Of the 48 patients, 28 had a textbook outcome after their surgery, a complex procedure to remove their tumors. The other 20 patients either had severe complications or were readmitted to the hospital – a complication rate similar to the national average. The team’s model, which combined the patients’ clinical characteristics with the Fitbit data, achieved significantly higher predictive performance compared with the American College of Surgeons National Surgical Quality Improvement Program surgical risk calculator.
Hammill has a master’s degree in aerospace engineering and was an aerospace engineer before going to medical school. He uses technology and innovation daily performing minimally invasive and robotic surgeries. Soon after he arrived at WashU in 2017, he met Lu, and they developed the plan to use wearable devices and machine learning to help predict and potentially improve patient outcomes.
Hammill said pancreatic surgery has a 40% complication rate due to the soft, crumbly nature of the pancreas, and surgeons have tried different techniques to improve that rate, but nothing so far has had much impact. The Fitbit provides an objective way to assess people prior to surgery over the longstanding method, which is to observe the patient walking, standing and sitting prior to surgery and at the first follow-up visit.
“Chenyang’s interest is in developing the machine learning and artificial intelligence that will allow us to make predictions about the patients who will do well with surgery or notice when someone is starting to have complications after the surgery,” Hammill said.
Lu and Hammill are teaming up again with another randomized trial looking at ways to avoid pulmonary complications after major abdominal surgery. They will provide patients with a Bluetooth-enabled, incentive spirometer, a device normally given to patients after surgery to exercise and strengthen the lungs, about two weeks before surgery to take a baseline of two markers of lung function as well as blood oxygen saturation. They will follow up immediately prior to surgery, the day after and on the third day after. That study also is funded by the university’s Big Ideas Competition, sponsored by the Healthcare Innovation Lab and the Washington University Institute for Informatics.
In another study, Lu collaborated with Thomas Bailey, MD, professor of medicine, and a School of Medicine team that monitored patients with heart failure who had been discharged from the hospital wearing a Fitbit device to predict readmission and death. Heart failure is one of the most common reasons for hospital admission in people over age 65. Readmission rates following discharge for these patients is about 25% within 30 days, and about one-third of those are readmitted for heart failure. This presents a need to continuously monitor these patients and note early warning signs to prevent deterioration and need for emergency room visits or readmission.
In the pilot study with 25 patients at Barnes-Jewish Hospital, published in ACM Transaction on Computing for Healthcare in March 2020, the research team found high levels of compliance with patients wearing the device and demonstrated the feasibility to identify patients at risk for deterioration using the data they collected from wearable devices.
While these studies have shown promise of wearable devices such as Fitbit, Lu’s team is continuing its research to overcome the obstacles posed by wearable data. The data these devices generate is fine-grained, meaning it gives minute-by-minute samples. In addition, the data is “noisy,” or cannot be easily interpreted by machines, and “lossy,” meaning there are gaps in the data, such as when a patient removes the device or it loses its charge. Another issue is that so far, the clinical studies on patients using wearables have had fairly small patient cohorts due to the critical nature of their health, which makes it challenging to apply traditional deep learning approaches that require large data sets.
“That’s why you have to extract features using engineering techniques,” Lu said. “How do we take this noisy, lossy data from wearables and extract robust and predictive features to generate something clinically meaningful and informative so we can actually predict something?”
Lu said interest in wearables is increasing because they can do much more to improve health than how they are currently used.
“We can scale this up to perfect the technology and broaden the scope so it can be used on larger groups of different types of patients,” Lu said. “I look forward to collaborating with even more physicians and surgeons to expand this work.”