MRI machines work, but why?
New research in Ulugbek Kamilov's lab begins to explain how it is that deep learning algorithms create accurate images — without a complete dataset
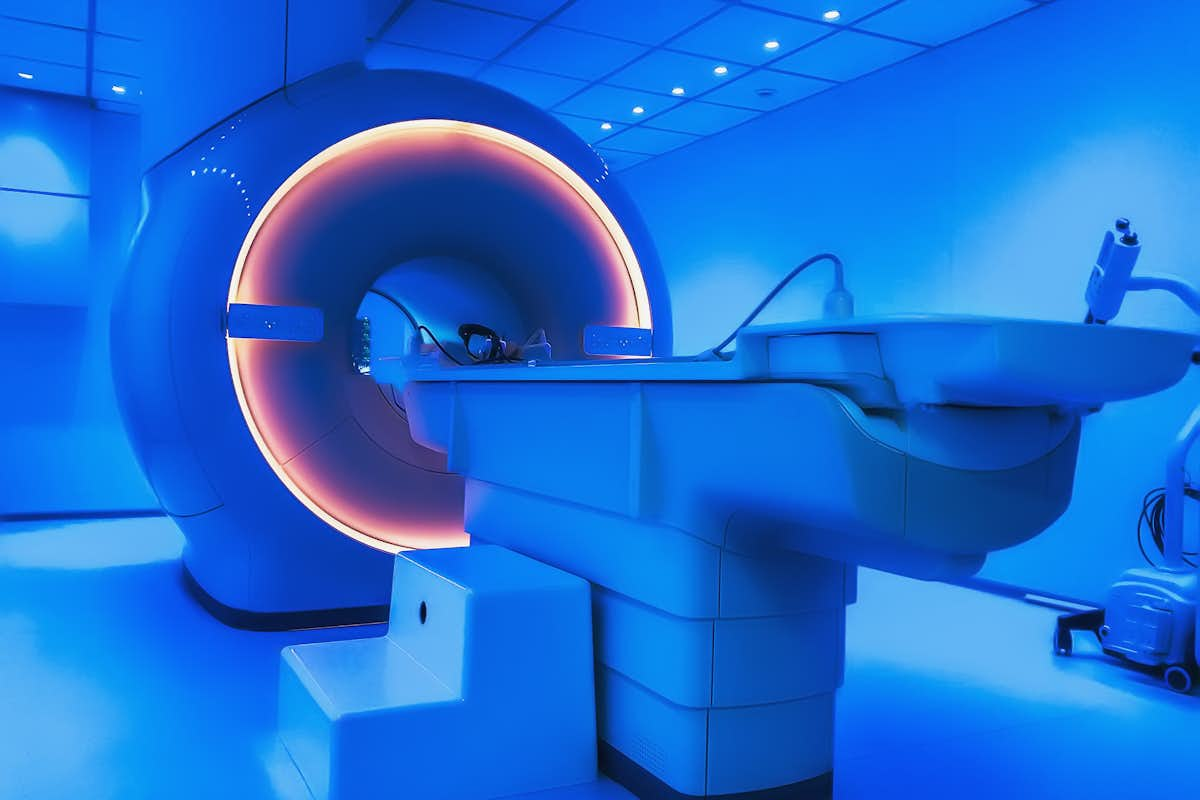
The speed of data collection in many kinds of imaging technologies, including MRI, depends on the number of samples taken by the machine. When the number of collected samples is small, deep neural networks can be used to remove the resulting noise and visual artifacts.
The technology works. Very well. But there is no standard theoretical framework — no complete theory — to describe why it works.
In a paper presented at the NeurIPS conference in late 2021, Ulugbek Kamilov, at the McKelvey School of Engineering at Washington University in St. Louis, and co-authors laid out a pathway to a clear framework. Kamilov is an assistant professor in the Preston M. Green Department of Electrical & Systems Engineering and the Department of Computer Science & Engineering.
Kamilov’s findings prove, with a few constraints, that an accurate image can be obtained by a deep neural network from very few samples if the image is of the type that can be represented by the network.
The finding is a starting point toward a robust understanding of why deep learning AI is able to produce accurate images, Kamilov said. It also has the potential to help determine the most efficient way to collect samples and still obtain an accurate image.
This research was supported by NSF awards CCF-1813910, CCF-2043134 and CCF-204629 and by the Laboratory Directed Research and Development program of Los Alamos National Laboratory under project #20200061DR.