Analyzing generative AI’s copyright crisis
WashU computer scientists developed a platform to evaluate the prevalence of intellectual property violations by code language models
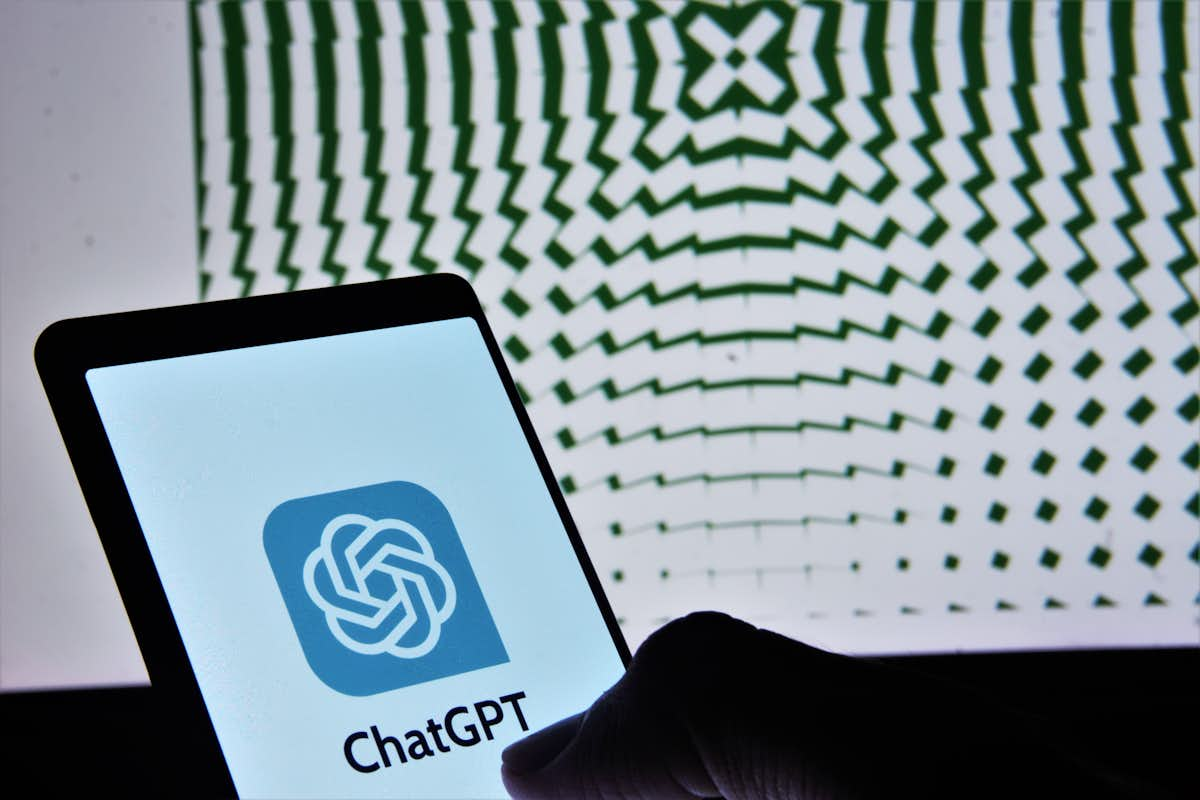
The recent explosion of artificial intelligence tools such as ChatGPT and Copilot have supercharged the assistance available to programmers. However, AI assistants may strip out comments embedded in code to convey copyright and attribution guidelines, leaving human coders none the wiser yet still on the hook legally for intellectual property infringement.
To combat this problem, computer science & engineering researchers in the McKelvey School of Engineering at Washington University in St. Louis have developed CodeIPPrompt, the first automated testing platform to evaluate how much language models generate IP-violating code. The team includes Ning Zhang and Chenguang Wang, both assistant professors; Yevgeniy Vorobeychik, professor; Zhiyuan Yu, a graduate student in Zhang’s lab and first author on the paper; and Chaowei Xiao, assistant professor of computer science at Arizona State University.
Yu presented the work July 23 at the International Conference on Machine Learning in Honolulu. Notably, the team’s analysis showed that copyright infringement issues are prevalent across state-of-the-art open-source models including CodeRl, CodeGen and CodeParrot, as well as in commercial products including Copilot, ChatGPT and GPT-4.
“We developed this tool to help people understand that if they’re using these large language models to help write code, there’s a good chance they might generate IP infringing content,” Zhang said. “As users, we have a responsibility to use AI ethically. That’s influenced by how we understand AI technology and the content it produces.”
Though CodeIPPrompt can’t say for sure if AI-generated code constitutes an IP violation – Zhang notes that issue is ultimately a legal question that will play out in the courts as cases are brought against the users of AI tools for copyright infringement – it can give users a risk score that indicates how similar generated code is to copyright protected content. Zhang anticipates that the tool will help guide the ongoing development of AI and point to potential mitigation strategies and other protections against IP violations in the future.
Yu Z, Wu Y, Zhang N, Wang C, Vorobeychik Y, Xiao C. CodeIPPrompt: Intellectual property infringement assessment of code language models. International Conference on Machine Learning, July 23-29, 2023. https://sites.google.com/view/codeipprompt
This work was supported by the National Science Foundation (CNS-1916926, CNS-2238635), Army Research Office (W911NF2010141), DHS (17STQAC00001-06-00) and Intel.