Consistency, trustworthiness in large language models goal of new research
Chenguang Wang plans to improve grounding ability with Google Research Scholar award
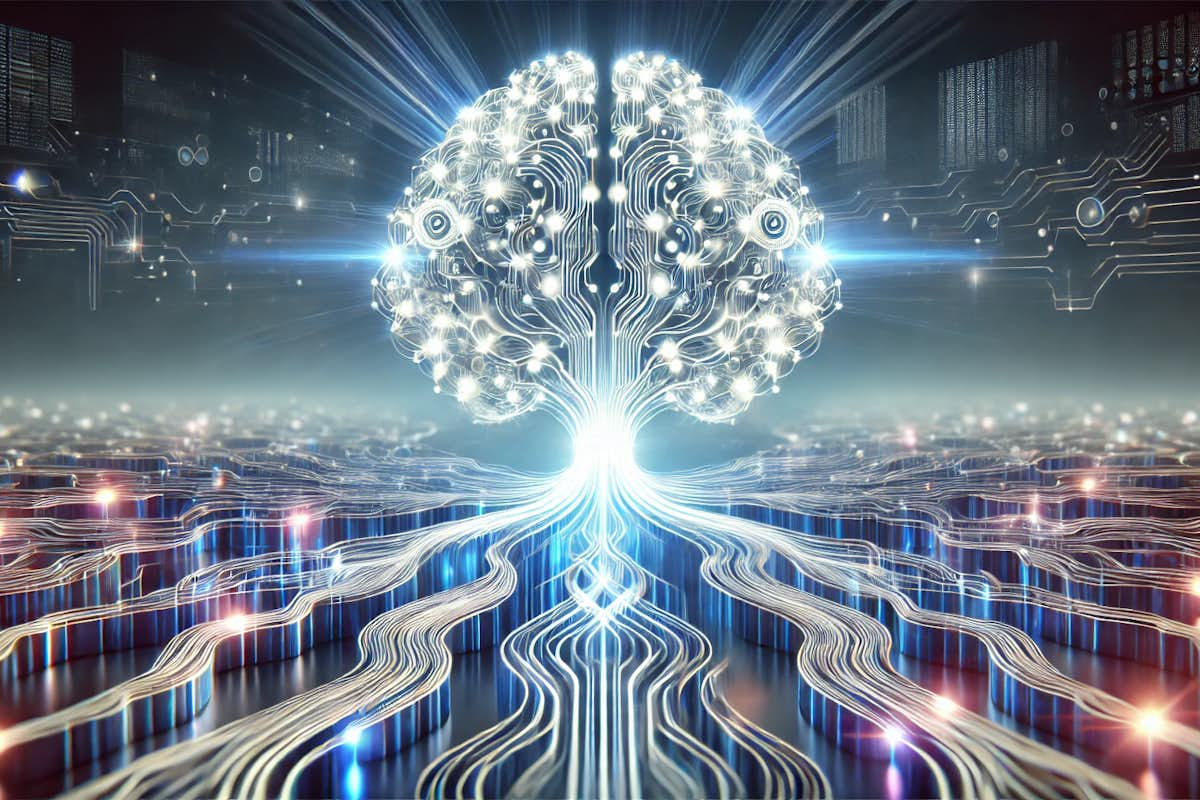
Large language models (LLMs), such as Chat GPT, are becoming part of our daily lives. The artificial intelligence models are trained with vast amounts of data to generate text, answer questions and prompt ideas.
However, one of the fundamental issues of these models is that they need more grounding, which refers to the ability to link their knowledge to real world facts. Failure to ground will lead to LLMs producing responses that are not consistent and unsafe, for example, misinformation and hallucinations. The recent White House executive order and open letters signed by thousands of researchers have highlighted its importance, especially in critical domains such as health care and science.
Chenguang Wang, an assistant professor of computer science & engineering at Washington University in St. Louis, plans to work with Google DeepMind researchers to improve the grounding ability of these models with a Google award titled “Before Grounding Responses: Learning to Ground References of Large Language Models.” This award provides a $60,000 gift, which supports early-career professors who are pursuing research in fields core to Google.
The goal of this research is to make LLMs more consistent, safe and trustworthy, by improving their grounding ability, which may lead to broader use in different areas. Wang plans to provide a new paradigm to ground LLMs to reference or source documents and focus on grounding references that LLMs used to answer questions. The proposed framework consists of a series of new learning techniques to help models use the correct reference when responding to user queries in different scenarios.
Wang’s lab has been working to improve the grounding ability of LLMs. His prior work, DeepStruct, is a 10B parameter LLM, which outperformed GPT-3 175B in 21 factual grounding-oriented datasets.