Engineering Depolarization with Jean Springsteen
Graduate student Jean Springsteen joins McKelvey’s Engineering the Future podcast to discuss her work at the intersection of computer science and political science
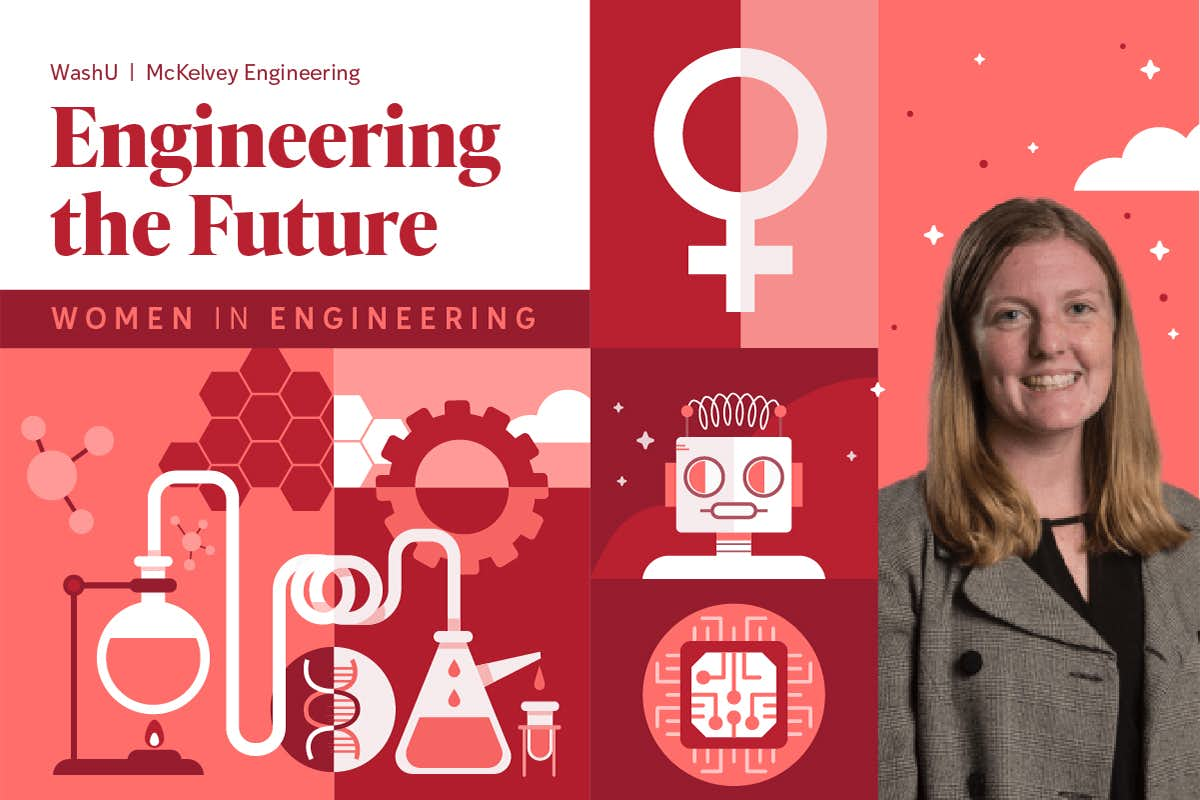
On this episode of Engineering the Future, Jean Springsteen, a graduate student in WashU’s Division of Computational and Data Sciences, offers a tantalizing glimpse into a future where social media isn’t so polarized. Tune in to learn how Springsteen and her advisers Will Yeoh, associate professor of computer science & engineering in McKelvey Engineering, and Dino Christenson, professor of political science in Arts & Sciences, are working to make democracy safer and Thanksgiving dinners less tense.
Jean Springsteen: Yeah, I think unfortunately the algorithms behind what we see when we log on to social media are really impersonal. They're interested specifically in showing me what they think will keep me engaged on a platform, right? They are interested in the profit and user engagement.
Shawn Ballard: Hello and welcome to Engineering the Future, a show from the McKelvey School of Engineering at Washington University in St. Louis. I'm your host, Shawn Ballard, science writer, engineering enthusiast and part-time podcast host.
Today I'm here with Jean Springsteen, who is a graduate student in Washington University's Division of Computational and Data Sciences, where she is working with Professor Will Yeoh, who is in computer science & engineering in the McKelvey School of Engineering, as well as with Professor Dino Christenson in political science, which is in Arts & Sciences. Welcome, Jean!
JS: Thank you for having me.
SB: All right, so I'm so excited to learn more about your research, which I understand is very intersectional, right? As you can sort of tell from that intro. You've got computer science; you've got political science. Working at that intersection, what are some of the big questions in that space right now? What really made you excited about answering those questions?
JS: Yeah, I think, you know, there are so many questions at the intersection, not just of computer science and political science, but of computer science and social sciences in general. One we hear about in the news a lot and like being on campus is, as AI becomes more accessible through LLMs like chat GPT, like the questions about the responsibility of training those models, right? That's very intersectional, and the place of AI in healthcare, education, all of these disciplines. That's probably one of the biggest questions right now.
Another big question, and the one I'm focused on, is at the intersection of computer science and political science. Social media companies use recommendation systems to show us when we log on to Facebook or Twitter or Instagram. They show us what is on our social media feed. That's the computer science side of it, right? But the impact of what we see on social media goes far beyond computer science, and that's the multidisciplinary. In political science, what impact does it have on our elections, on people's ideology?
So, that is a pretty big question at that intersection, and because social media impacts the way we interact, the way we think, you know, what we see on social media impacts what we talk about at Thanksgiving dinners.
SB: Right, unfortunately.
JS: Right, it's not always a good thing. So, because it's so real world and has an impact on how we interact with the people closest to us, I think that's what draws me to questions like this.
SB: So, thinking about those sort of large language models, right, and the algorithms that are behind, you know, your Google search or what's on your social media feed, those feel very impersonal and on the computer science side. I love how you talked about bringing that toward a more personal impact. Can you talk about that tension a little more? Is the algorithm side as impersonal as I think? Yeah, weigh in on that.
JS: Yeah, I think unfortunately the algorithms behind what we see when we log on to social media are really impersonal. Social media companies aren't trying to show us, you know, my new niece and nephew, right? Like they're not interested in showing me that. They're interested specifically in showing me what they think will keep me engaged on a platform. Right, they are interested in the profit and user engagement.
And so that does make it pretty impersonal in terms of they might be showing me incendiary content or misinformation because, you know, their algorithms, through their machine learning techniques, their artificial intelligence, they're learning that that's what drives user engagement, not necessarily what I as a user want to see when I log on.
SB: Yeah, and that really just bums me out, I guess, as you're telling me this, because it feels like social media should be connective, right? Like ‘social’ is right in the title. It should be connective in some way, and of course it's made by humans for other humans. And yet, like what you're finding is that that's really been reduced down to sort of the bottom line, the marketing, what engagement is all about. And yeah, that is just a bummer. That's my takeaway from that.
JS: Yeah.
SB: Okay. So, you've got that kind of like, that feels like a bit of bad news. But you're maybe, I hope, putting some more good news in there with the social science side of it. So, as you're analyzing what's going on with those algorithms that really are not caring about the human experience, how are you able to sort of work with that in a way that is influenced hopefully more to the human side with social sciences?
JS: Yeah, so the algorithms, they're focused on user engagement, but we are interested in seeing how polarization is seemingly increasing. What can we do from the algorithm side to reduce that polarization? So, instead of having algorithms focused just on user engagement, what if we find some metrics such as like extreme policy, like how do people feel about policy? How do people feel about political candidates from their party and the other party? And using those as metrics instead of just user engagement.
And so, if we're designing algorithms to reduce polarization that way, hopefully it's a little more personable and we see, you know, our recommendation systems would bring in less polarizing content and maybe more content that we'd actually prefer to see on social networks.
SB: Oh my gosh, I would love to see that.
JS: Right?
SB: I feel like we all would.
JS: Sounds pretty idealistic.
SB: It does. So yeah, I love that idea. This sounds like a really promising project, especially as we're, you know, the election is right around the corner. So, this is on a lot of people's minds right now. How, I guess, like what is the outlook on that, right? Does this seem – I know you're sort of early in your work, you're still on this project, right? It's not done yet. But what sort of signals of possibility or of hope are here for this project? Like how are you feeling about it so far?
JS: I think the biggest signs of hope are that this is something that a lot of people care about. So, there's a lot of research to pull from. A lot of people are working on this project in computer science and in political science. We're like one of many different projects, different approaches to reducing polarization. So, I'm hopeful that our project is on the right path and we're going to learn some ways of polarization reduction. But we're far from the only ones attempting this, which I think, you know, personally provides me a lot of hope that maybe we're not, you know, just off on the wrong path indefinitely on social media.
SB: Okay. That is, that is hopeful, right? You know, I don't want to get too like, I guess like, pollyannish about this. Like these social media companies are very big and very sort of set in their ways and making a lot of money on those ways. So that's, that is an uphill battle for sure. But, you know, researchers like you in, you know, both computer science and social science are taking this on. How, how do you, how do you decide to sort of tackle that? Right? Sort of what brings you from, you know, your math background, your computer science background to putting this together with social science? Because this seems like really rewarding work. But, you know, not easy and not the most obvious pathway.
JS; Yeah. And especially not easy in terms of data restrictions. Like as social media companies are becoming more influential and growing, the lack of data access for researchers like us is complicated. But that I think is part of the reason, like my math background, computer science background, we can kind of come up with ways to circumvent that and, you know, run simulations and do user surveys to get our own data instead of relying on the access of social media data, which is decreasing.
SB: Even with the restrictions that, you know, outfits like, you know, Facebook and Twitter and Reddit, you know, that you hear about, they're not giving access even to researchers as much as they were before. Even with those restrictions, you're able to get a clear enough picture to be able to see, you know, what is probably or likely happening on there.
JS: There's no way to know for sure, right? Because that data is restricted, we can't compare it and say how close did our model actually get. But that's why we make informed modeling choices. We talk to other researchers. We pull in the political scientists and say, you know, what are you seeing? How can you inform our modeling choices as well?
SB: Okay. So even something like that modeling problem seems like more, I was reading that as more purely like computer science, but that is also informed by that social science aspect. And really that's coming in from the ground up for your work.
JS: Yes, especially if we look at like polarization metrics, right? What we think of as a typical polarization metric might not be what political scientists are actually thinking of as polarization metrics. So, pulling that in and actually making sure, right, when we build a model that we're looking at the right things and using it in a way that would be interesting across disciplines.
SB: Can you give me some examples of that? So, I can sort of consider like, you know, a polarization metric might be looking at like somebody liked, you know, extreme content or something. Is that, you know, is that one of them? Yeah, what do those look like, and sort of what would a computer scientist pick out as polarization metrics versus a social scientist, and like how close are those?
JS: In terms of, for example, climate change policy, you might have two very different groups who have very different preferences on what they would like to see or not see happen, right? That's, you know, specific policy that's on an ideological spectrum.
Affective polarization isn't so much about policy or ideology. It's more based on party. It's not “I dislike this political candidate because of their stance on climate change policy.” It’s “I dislike this candidate because they belong to a different party.”
SB: Okay. So that's affective with a “a.” So, it's like your heart says, “no, I don't like that party.”
JS: Yes.
SB: Okay.
JS: And so that, we did not really think about incorporating that kind of polarization into our models until we talked to faculty in the political science department who said, you know, this is a type of polarization we're interested in, that we see increasing affective polarization. Can we, you know, put that in your model? Can we figure out how to model that? And so that's how different, you know, disciplines can really change how you are focused on your models.
SB: Okay. And so, when you're tracking, you know, affective polarization on social media, it's not the, you know, the person liked this extreme post. It's something else. What does that look like in social media data?
JS: Yeah, I think we can all think with scrolling through social media. Sometimes you're not seeing someone post about a certain policy. You're seeing, you know, name calling. You're seeing “this Democrat,” “this Republican” and like focusing the party, the group someone belongs to instead of what their ideology looks like.
SB: So, you're seeing this increase over time, and your colleagues in political science are saying this is increasing, we're seeing more of this affective polarization, we need to take that into account. How does that impact like what comes next? So, you're putting this into your model, into your simulated data, and then you're also doing user surveys. Is that yeah, take me through the rest of this pathway here.
JS: Yeah, so our next step is to run some user surveys and see if we can take or find aspects of social media posts that kind of elicit these affective responses. When we open social media, there are so many like granular factors that maybe we don't even like consciously think about when we're seeing and scrolling, right. We see who is posting it, and we're reacting to that a lot. But there are so many other factors, just the order in which you see posts, the who sent it to you or who reposted it. Like there are so many smaller factors that also change how we how we respond and how we internalize what we see.
And so, through the user survey, we hope to be able to isolate like some of those smaller parts and say if we, you know, it's very lab setting almost, so like we specifically change like these one or two small factors, does that change how people react to the post in terms of, do they report higher levels of animosity towards the person or the party posting it?
And so hopefully through the user survey – because we don't have access to the social media data we want – through the user survey, hopefully we can isolate some of these smaller things and use that as lessons and say, you know, if we change these small parts of our social media posts, maybe we can have less polarized responses from the social media posts.
SB: Okay, so that is all, it sounds enormously complicated. Lots of tiny factors playing in both sort of that influence behavior, and then behavior in turn influences the next thing you get in the network.
JS: Yes.
SB: So, it sounds like, you know, obviously like bringing a lot of work to bear in the computer modeling, but as well as those insights from political science. I want to dig into how those, you know, fortuitous connections and collaborations come about through the Division of Computational and Data Sciences, DCDS, and how you got involved with that. Can you tell me a bit about the work? Is it all like this or, you know, what's it look like over there?
JS: Yeah, DCDS is great in that the whole goal of the program is to have this interdisciplinary work. So, we can use data science to answer questions in political science, which is what I'm doing, but also in psychology and public health and all of these fields. And so, I was interested in doing interdisciplinary work and DCDS is the perfect spot and not only encourages it but forces it as part of the program.
SB: Yes, yeah, yeah. It's like, come in here, you know, if you love this, you know, or else, right?
JS: Yes.
SB: No, I'm sure it's a very supportive environment and you have, you know, two faculty really working with you on that. So, these things have to work together in a way that's cumulatively supportive. How does that work? How do you navigate having, you know, your adviser, you know, Will Yeoh in computer science here in McKelvey and then also working with Dino Christenson in political science and Arts & Sciences? Obviously, we have, you know, shared goals across our schools and that's great, right? We're all here at WashU together. But I could imagine there being sort of, you know, competing priorities, right? Are those difficult to navigate, and how do you navigate them?
JS: I haven't found them very difficult to navigate up to this point because I think the biggest thing is when we are working on this project, we all have the same goal in answering this question. And I think, you know, personally, we all understand that our expertise in computer science or political science might not be the only way to answer that question. And it's not, “I know this is one way to do it.” I think we're all kind of interested in hearing what would someone else approach this question with? What background would they approach with?
Because that gives us not only more tools when something doesn't work out, when our first step is wrong, and we go back, at least we have a wide pool of expertise to pull from, but it also helps give more complete answers.
SB: So, you've told me a lot about this project you're working on right now and sort of how you're considering different kinds of polarization and ways that you can measure that, track that on social media. As you're thinking about interventions to reduce that polarization, what is the, sort of, what's the outlook, what's next, right? Like, sort of, you know, I know this is probably several years in the future at best, but what are sort of the goals, like, longer term for you?
JS: Yeah, so the goal is, once we learn, maybe if there are parts of social media posts that create, you know, extreme or polarized responses, how can we balance that with user engagement metrics?
There's a whole body of literature that talks about what makes people, you know, stay on social media, like what kind of posts keep people engaged. And so, if we can add to that, well, what kind of posts make people more polarized or what kind of posts make people less polarized more specifically, how can we balance that user engagement with polarization?
So, for us, it's not enough to say, we think this is what will make people less polarized because the social media companies, they'll hear that and say, great, we care about user engagement, we're not interested in that. So, the next step is to try to balance those things. Can we find posts or, you know, sets of posts that keep user engagement high that people want to see and interact with, but that maybe are less extreme or less misinformation on social media?
And hopefully then, social media companies will be a little more receptive and say, okay, maybe this is something we can implement because our user engagement metrics, our profit levels, aren't impacted as much.
SB: Okay. And how would that, you know, finding those sets of posts that have those patterns of maintaining high engagement with low polarization, how would that sort of get incorporated or put into practice by social media companies? Would that be like a model for them? Those patterns would suggest a way to change the algorithm or to, I don't know, I'm sort of imagining, like, how would that be able to change what appears on social media platforms, right? Because those are user created and, you know, it's the Wild West out there, right?
JS: Yeah. So, I think there would be multiple ways to, you know, implement some of these recommendations. We are focused on the recommendation systems, those algorithms, because that's how, you know, if we see posts with X and Y qualities elicit less polarized responses, right, how can we put that into a filtering strategy into that recommendation system and say, all right, maybe let's focus on X and Y, and if Y happens to be a metric that increases user engagement, right, like that's the kind of thing we want to see. And then in those recommendation systems, we can focus on, you know, those features, right. Show people posts with X, Y features.
SB: I see. Okay. So, the implementation is you found these patterns that, you know, achieve the engagement, but not the scary polarizing parts, right, and then say to social media companies, look, this won't hurt your bottom line, but you can promote these things that not only don't hurt your bottom line, but like don't hurt, you know, people's Thanksgiving dinners or democracy or, you know, these things that we like.
JS: Yeah, that's the idea. That's the hope, right?
SB: So, you mentioned that your background is not in computer science. What is your background, and how did you sort of get to be doing this intersectional work?
JS: Yeah, so my background is in math and economics. I've always known, like, doing things with numbers, doing like computational work, like, that's where I want to be. That's what interests me. And, as I did more and more math, it became less and less tangible, like in the real world, talking to my parents, my friends who ask, what are you doing? It's not very tangible to explain to them, right?
And then as I'm figuring out what I want my research career to look like, right, this is in 2020 where so much happened in the world that was really tangible, right? Like real world effects. We’re at home all the time. We are watching the news, and it's just, it made me want to do something tangible.
SB: Based on your desire to make those tangible impacts and seeing how that could come through in, you know, implementation on social media algorithms and promoting those things that will not tank, you know, Thanksgiving dinner table conversation. Can you talk to me a little bit more sort of about the stakes of that, right? They seem very high, but I guess I want to get a sense from you as an expert sort of from what you've seen in patterns on social media and the social science aspects of that. Like how actually scary and how big of a problem is this polarization basically? Like what are the stakes?
JS: Yeah, I think, you know, we hear about it for a reason, right? The actual real world impacts we've seen in the January 6th insurrection at the Capitol, right? Like that is a real-world consequence of what happens on social media, polarization that comes from social media.
And so, it is scary in the sense that we seem to be headed down this path and if, you know, no one does anything to check the direction we're going, you know, what other real-world consequences are we going to see? It's not just, you know, fighting on Twitter, fighting on Facebook. It's, you know, political violence. It's decreasing trust in our elections, right?
So, it is scary in the sense that it's real, right? We're seeing these impacts. The hope I have is that a lot of people are working on this, right? We have people who want to make this better, and I think in general people are not happy with how polarized social media has gotten. So, in general, there's hope that, while it is scary now, hopefully, you know, our research but an entire body of research can help this, help quell this kind of polarization.
SB: Okay. So, you have given me a lot to chew on and some more hope, I will admit.
JS: I hope there's more hope.
SB: There is more hope. So, all that aside, though, I want to ask you, maybe the most important question, Jean, what have you been enjoying lately in terms of media? Is it a TV show, book, movie, podcast? What have you got? What if we need a break from all this polarization, what do you recommend?
JS: That is a tough question. Well, my go-to TV show recommendation, especially in the fall, is Gilmore Girls. It's, you know, 20 years old at this point, but anyone I talk to, that's what I will recommend. It's cozy fall vibes.
SB: Fall vibes, yeah!
JS: It definitely takes your mind off of, you know, the real world as it's, you know, ideological Connecticut. But that's always my recommendation.
In terms of reading, I recently read Beartown and its sequel, Us Against You is what it's called. And I really enjoyed those. They’re by Fredrik Backman. It's a small-town hockey team and, you know, what happens when a sports team is kind of the focus of a dying town. So, I really enjoyed those books. There's one more in the series that I'm looking forward to read soon.
SB: Those do sound very cozy as we move into these, like, cooler months. So, thank you so much for that. And thank you for joining me today on the podcast. I have learned a lot, and I am very looking forward to you changing social media for the better. So no pressure! You and Will and Dino have your work cut out for you.
JS: Definitely. Well, thank you for having me.
[music]
Click on the topics below for more stories in those areas
- Undergraduate Students
- Alumni
- Outreach
- Podcast: Women in Engineering (2024)
- Diversity
- Graduate Students
- Women & Engineering
- Computer Science & Engineering