How artificial intelligence can facilitate the literature review process in wastewater-based epidemiology
Fangqiong Ling’s team compares the performance of different generative pre-trained transformer (GPT) models in facilitating literature screening for wastewater-based epidemiology
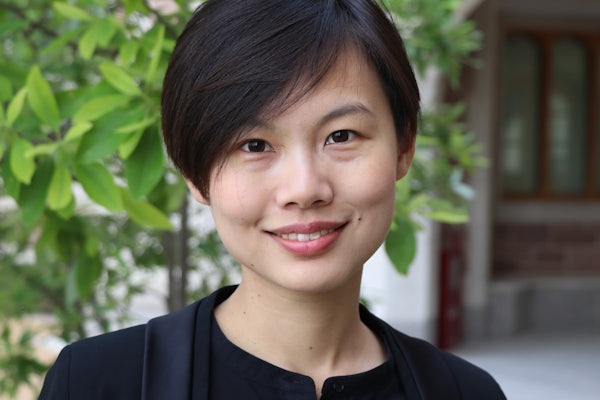
Wastewater-based epidemiology is an emerging field that tracks community-level health statuses by analyzing untreated wastewater. As the volume of literature in wastewater-based epidemiology has rapidly expanded, there is an increasing need for effective ways to review vast amounts of literature. Systematic reviews and meta-analyses are useful methods, but the literature screening processes can be labor-intensive and time-consuming. This highlights the growing need for innovative solutions to simplify these processes, transforming the challenge of handling large datasets into an opportunity to enhance research efficiency and improve public health responses.
Recently, a new research study at Washington University in St. Louis evaluates the potential of artificial intelligence (AI) to assist in the literature screening process in the field of wastewater-based epidemiology. Fangqiong Ling, assistant professor of energy, environmental & chemical engineering in the McKelvey School of Engineering, explores how different versions of GPT models can be applied to identify relevant studies and filter out unrelated ones.
“The wastewater-based epidemiology field experienced a rapid growth in literature since the COVID-19 pandemic,” Ling said. “In talking with colleagues from computer science, they suggested we look into large language models, which may help us automate the process of screening the papers for qualifying studies.”
Ling’s study focused on comparing different models of GPT to see which could most effectively screen literature based on specific criteria, such as accuracy, precision and recall. Among all criteria, recall was given particular emphasis. “We anticipate the literature screening by models to precede that by human researchers and it’s helpful for this step to be more inclusive,” Ling said.
The study found that the GPT models could quickly identify relevant studies with impressive accuracy. GPT models were given abstracts or methods from research papers and tasked with determining whether the paper met specific criteria, such as containing original data or relevant sampling locations.
The models were evaluated using two approaches: a text completion approach and a classification approach. In the text completion approach, the model was asked a direct question, like "Does this paper contain original data?" While some responses were restricted to “yes” or “no,” this was not always required, as the study explored different parameters, including variations in input and response format. The classification approach, one of the fine-tuning functions of the OpenAI tools, on the other hand, involved fine-tuning the model using human-labeled examples to classify papers as relevant or not based on the same criteria.
The results showed that GPT-4, GPT-3.5-turbo, and fine-tuned Davinci models achieved recall values near 1.00, surpassing the standard of manual screening (Recall = 0.95), and demonstrating exceptional accuracy in identifying papers with original data. This level of performance was achieved with each abstract processed for less than 1 cent and in under a second.
Kaseba Chibwe, the first author of the study, highlighted the value of AI in enhancing research processes.
“This is a great demonstration of how AI can be used as a tool, not a shortcut, which will lead to greater efficiency in research synthesis,” Chibwe said.
Looking ahead, Ling and her team are focused on further advancing the field of wastewater-based epidemiology. They aim to derive more accurate insights from wastewater data to contribute to public health improvements and the development of sustainable cities.
More about Ling’s research can be found in ACS Environmental Au published Dec.3, 2024.
Chibwe K, Mantilla-Calderon D, Ling F. Evaluating GPT models for automated literature screening in wastewater-based epidemiology. ACS Environmental Au, Dec. 3, 2024. DOI: https://doi.org/10.1021/acsenvironau.4c00042
This work is supported by the National Science Foundation CAREER Award (2047470, F. L.) and the National Science Foundation Graduate Research Fellowship Program (Grant No. DGE 2139839, K.C.).