Novel learning method proposed for generative AI in challenging environments
Yevgeniy Vorobeychik, Ning Zhang and William Yeoh receive $1.5 million DoD grant to enhance multiple facets of generative AI
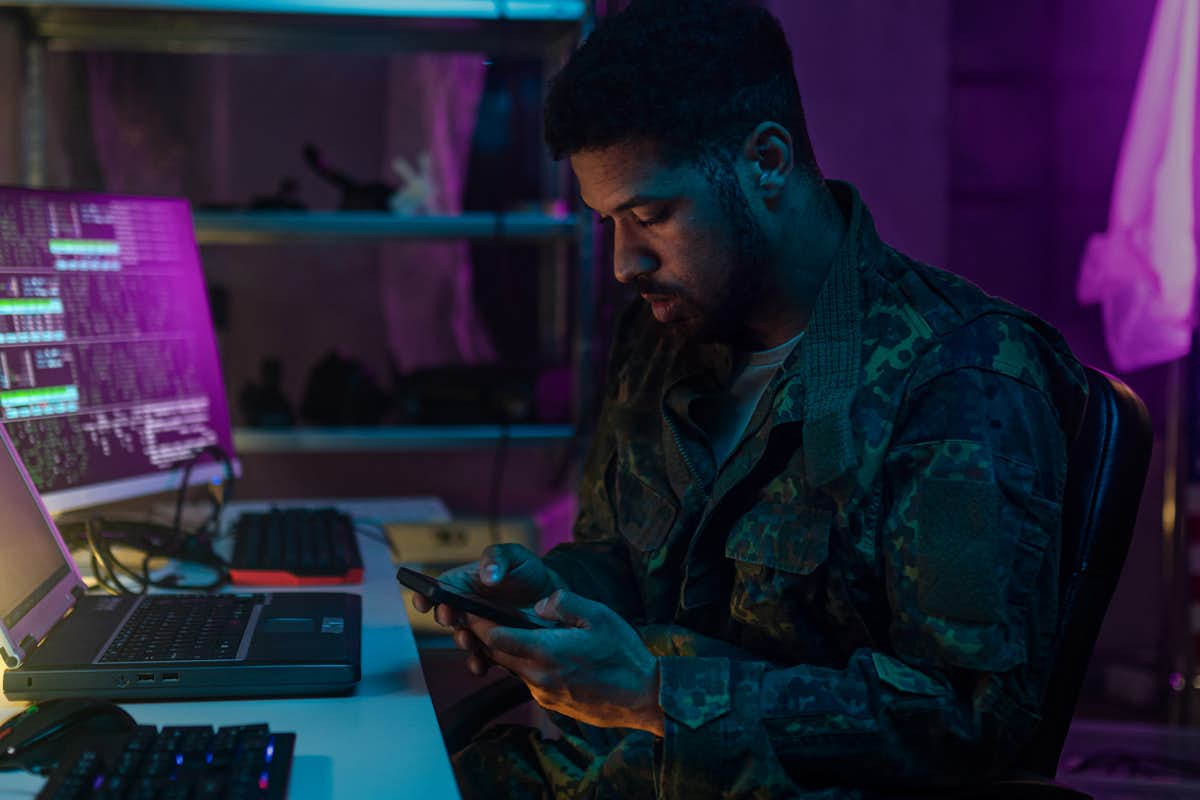
In the world of generative artificial intelligence, generative adversarial networks (GANs) are simultaneously forgers and detectives identifying forgeries. GANs use artificial neural networks to generate new data – the forger – and evaluate that data to determine if it’s real or fake – the detective. The forger and the detective train against each other until the GAN’s created data is indistinguishable from the real thing. GANs can create or enhance images, sound and video based on incomplete or low-quality data, making them especially useful in challenging environments, including inaccessible, remote or embattled settings.
Improving the performance of GANs in such challenging environments is the focus of new research led by Yevgeniy Vorobeychik, professor, and co-investigators Ning Zhang and William Yeoh, associate professors, all in the Department of Computer Science & Engineering in the McKelvey School of Engineering at Washington University in St. Louis. The team received a three-year, $1.5 million grant from the U.S. Department of Defense (DoD)’s Office of Naval Research to support their work on federated learning for generative AI.
Traditional GAN models rely on centralized data repositories, making them impractical for many DoD applications where data is stored on remote devices in dynamic environments. Federated learning, a decentralized framework that enables training models across distributed devices without the need for data sharing, has gained traction in some areas of machine learning. But its application to GANs, especially in the complex and varied environments encountered by the DoD, is still largely unexplored.
“Federated learning allows us to avoid the risks associated with centralizing sensitive data while still enabling effective machine learning,” Vorobeychik said. “However, we must move beyond current models that assume synchronous updates and uniform compute capabilities. To make federated generative AI practical in military settings, we need to account for the unique challenges of DoD environments, including communication constraints, highly sensitive data and rapidly changing conditions.”
Vorobeychik, Zhang and Yeoh propose a novel, comprehensive approach to federated generative AI. By integrating learning algorithms in their system design choices, the team aims to maximize learning efficiency and user participation, even in difficult conditions with unreliable communications and varied data streams. As part of the project, the team will use a battlefield federated learning testbed to evaluate and refine its proposed methods with particular attention to communication efficiency and security in real-world, mission-critical scenarios.
Beyond improved capabilities for military applications such as remote surveillance, reconnaissance and mission planning, this research has wide-reaching implications for civilian use. By enabling secure, decentralized learning on remote devices – from cell phones, where centralizing data is impractical, to health information systems where privacy is paramount – the research strives to unlock the full potential of generative AI without compromising security, privacy or efficiency.