Kantaros to unlock new possibilities for autonomous systems
Yiannis Kantaros plans to advance the safety, efficiency and transferability of AI-enabled autonomous robots
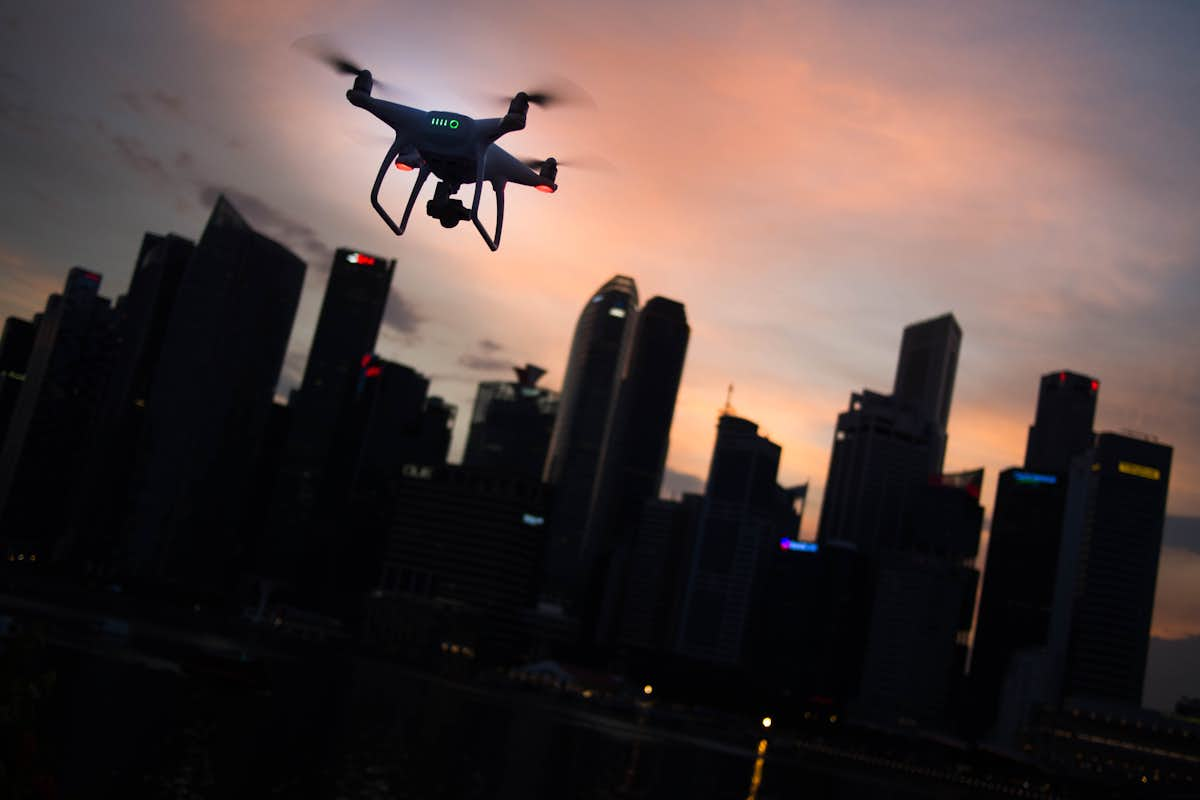
Deep reinforcement learning has received a significant amount of research attention recently as it enables autonomous systems, such as autonomous cars or drones, to make decisions in real time. However, researchers are still grappling with the computational demands of learning such controllers as well as the need to guarantee their safety and transferability into novel environments.
Yiannis Kantaros, assistant professor of electrical & systems engineering in the McKelvey School of Engineering at Washington University in St. Louis, has received a $413,694 grant from the National Science Foundation to address these challenges. Kantaros aims to develop data-efficient machine learning methods that can create safe and verified controllers that allow autonomous robots to satisfy complex mission and safety requirements that may be different from the ones used during the robot’s training phase.
“Artificial Intelligence for control and perception has revolutionized robotics as it has enabled a wide range of autonomy applications,” Kantaros said. “For instance, aerial and ground robots can now be used for urban delivery tasks or for exploration of unstructured environments thanks to recent advances in machine learning. I’m really excited to develop machine learning methods that have the potential to unlock new possibilities for autonomous systems.”
Kantaros said the top challenges in developing controllers that can accomplish such complex tasks are high computational demand, massive data requirements, and lack of safety and transferability guarantees. To address these concerns, Kantaros will investigate how to actively collect the minimum amount of data required to learn controllers that achieve desired performance requirements. He will also examine under what settings the learned controllers can be safely transferred to new tasks or environments that are new to the robots.
“The algorithms developed in this project will have several autonomy and safety-critical applications,” Kantaros said. “For instance, consider robots tasked with a surveillance or environmental monitoring mission. Such a mission might require the robots to visit certain locations of interest in a forest to collect temperature measurements to prevent wildfires. This robot task can change abruptly due to unanticipated events, such as wildfires or the presence of humans, or to changes in the locations of interest. The proposed research will enable the design of controllers that can safely adapt to such changing conditions in real-time.”