Engineering AI for Health with Chenyang Lu
Computer scientist Chenyang Lu kicks off a new season of Engineering the Future with a discussion of how AI is impacting human health
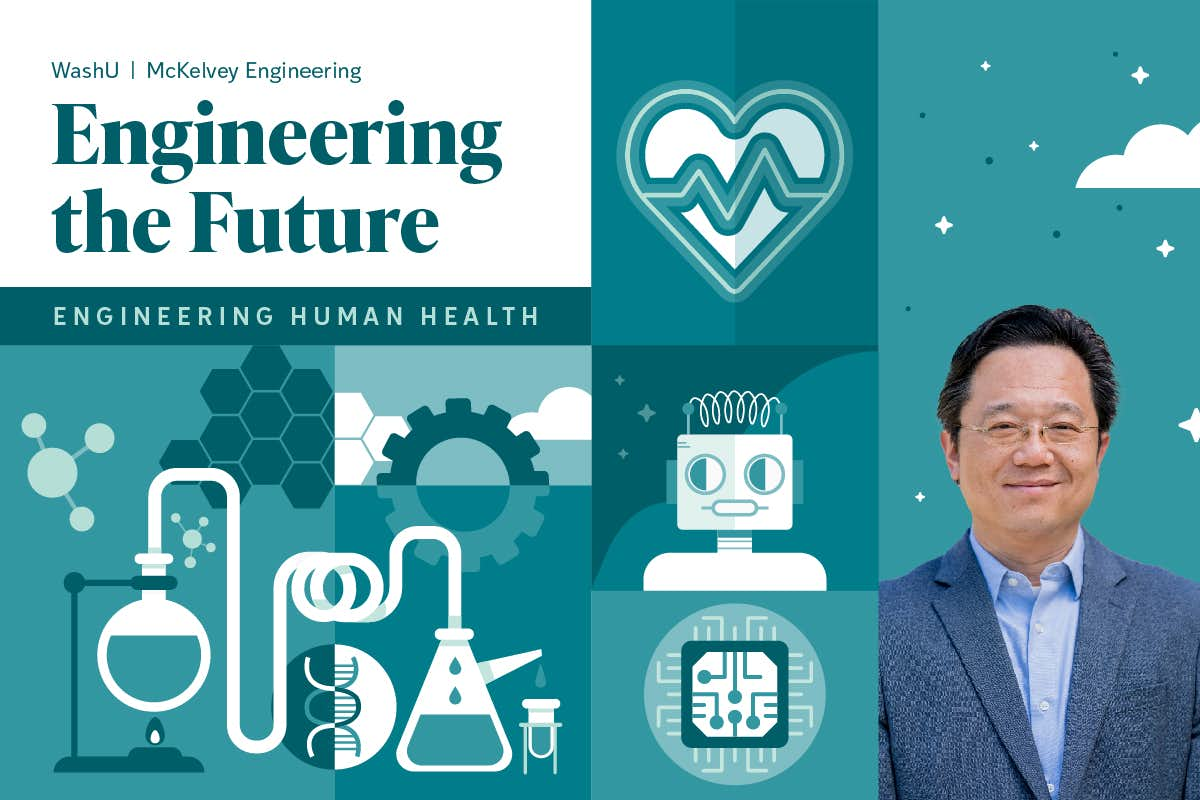
This season on Engineering the Future, our theme is Engineering Human Health, and we’re starting with a look at how AI is changing the health landscape from hospitals to public health policy. Chenyang Lu, the Fullgraf Professor in the Department of Computer Science & Engineering, discusses his efforts to bring together collaborators from across WashU as director of the AI for Health Institute. Lu also shares the latest research from his lab on wearables and how AI can make personalized health recommendations.
Chenyang Lu: We have AI looking at clinical images, and we have radiologists looking at the same images. They discover different things, and how do they work together in the most effective fashion so that collectively you reach the best decisions?
Shawn Ballard: Hello, and welcome to Engineering the Future, a show from the McKelvey School of Engineering at WashU. This season our theme is Engineering Human Health. I am Shawn Ballard, science writer, engineering enthusiast and part-time podcast host. Today I am here with Chenyang Lu, who is the Fullgraf Professor in the Department of Computer Science & Engineering, as well as the director of WashU's AI for Health Institute. Welcome, Chenyang!
CL: Glad to be here.
SB: It's so great to have you. I want to jump right in with your latest big venture, which is the AI for Health Institute. That launched in October 2023, still relatively new, so people may not know, what is the AI for Health Institute, and how did it come to be?
CL: At the AI for Health Institute, the mission is to bring together AI experts and health researchers together to tackle important health problems with advanced AI. It came about when we realized there's this gap between AI in engineering versus AI that's being used in health, right, be it health care or public health.
In a sense, on one hand we have these brilliant health researchers trying to take advantage of the data and apply AI models and approaches to solve their problems. Because naturally they are not trained AI experts, right, so they are using relatively, you know, sort of textbook, basic AI techniques to solve these problems. Sometimes it works, and sometimes it gets difficult when the data is complicated and the application is hard.
On the other hand, on the side of, you know, engineering and AI, right, this is of course an explosive field in computer science, and new techniques are being developed every year, every month, right, you see a lot in the media.
SB: Feels like practically every day!
CL: Exactly, but, you know, these truly cutting-edge AI techniques are scarcely applied to health because of the natural division of different expertise and different communities and different campuses sometimes. So the idea of the, we felt like this is a huge, sort of, missing opportunity, right, for mankind and for health research, in the sense we have all these powerful AI that could really solve some of these really important and challenging problems in health, but we need to bring people together to do it, right, so that's how the AI for Health Institute came to be.
SB: Gotcha. What are those sort of big problems or questions that you're addressing with the advanced AI?
CL: Yeah, so there's a lot of really important problems that we need to work on. There are several examples: for example, depression. According to the WHO, over 280 million people have depression. To make matters worse, over 50% of them are either not diagnosed or not treated, so we have a huge under-diagnosis problem.
The reasons are fairly clear. We have a scarcity of mental health professionals, and also people are generally reluctant. There's a certain barrier to go and see a psychiatrist for the depression problem.
So, there’s got to be a better way, right, to screen for depression, to detect potential depression earlier. So, one of the research projects we are doing is to use wearables combined with AI to detect depression. Think about Fitbit wristbands. It would be great, right, if you just look at the Fitbit wristband data and then be able to detect if this person has higher risk for depression.
This is not science fiction, right, in the sense that if you think about what these wristbands measure, they measure activity step count, which is widely established in the mental health literature to be associated with depression.
SB: Oh, I didn't realize that.
CL: Right, and also sleep patterns, right, is widely known to be associated with all kinds of mental health conditions. Heart rate, you know, heart rate patterns are associated with our mental conditions as well. So, to be able to go from these data, that's being unobtrusively collected on a daily basis, and then this is where you really need AI. You need deep learning to discover these really complex patterns and detect the person with higher risk for depression, and hence they should seek help.
Essentially, deep learning is extremely good at identifying the complicated correlations between these different characteristics and features of this data with the health outcome of interest.
SB: That sounds like there might be something sort of sticky there with patient privacy when you were talking about, you know, these undiagnosed cases or perhaps incorrect diagnoses. How much do you have to worry about those questions in your data sets, like is this data accurate? Is this data being ethically sourced? All those kinds of questions we hear about AI broadly, those of us who are using things like large language models. They're harvesting all this data, and a lot of people don't like that. It seems perhaps even more potentially tricky with medical data.
CL: Right, yeah, these are, you know, very, very important questions. In that particular case of the depression study, this was based on this large-scale study that happens at NIH. It's a project called All of Us, the program. The idea is to collect over a million people's data and use that data to drive data-driven approaches to precision medicine.
So, all these data are carefully safeguarded in a cloud platform hosted by the research program. Obviously carefully anonymized, so that you can only get this data by working on their platform, so it's kind of a controlled environment that you have to work with this data. But, more broadly, certainly that's very true that we have to be very mindful and carefully protect privacy of these data.
So, generally speaking, there are two-pronged solutions. One is regulation. There clearly, you know, you need to have a lot of regulation, so to safeguard patient privacy, and that regulation is evolving because of the arrival of AI. There's a lot of new challenges. And then there's technical solutions as well. There are solutions that are specifically designed to train the model in being able to do inference without the potential of revealing individual data.
SB: You talked about the Fitbit data and depression. What other kinds of big projects are going on under the auspices of the AI for Health Institute?
CL: Clearly, we're building the community, and we are connecting faculty from different areas to pursue large research initiatives, and some of them are ongoing right now, involving multiple departments across schools to pursue these really high-profile health care problems. Such as, how do you make your machine learning models stable over time as you redistribute these models in different locations and different communities and different hospital environments? How do you get your AI models for health care applications to maintain their peak performance and self-detect its performance degradation and self-correct its behavior? These are vital problems, as we see AI start being deployed in health care applications.
And also, you know, we want to encourage and enable many more in the community to work together on AI for Health projects, so that's why, you know – a little plug here – we are launching the AI for Health seed funding program with a deadline in March. This is in collaboration with the Here and Next strategic initiative of the university. So basically this has to be multi-disciplinary teams employing advanced AI technologies to important health care problems.
SB: So this is really like a whole new way of doing things.
CL: It's a new era of AI for Health.
SB: Yeah, absolutely. Thinking about people who might be joining these kind of collaborations, who you'd be reaching out to. The big ones I think of, of course, are in computer science, people who work with AI directly, and then, you know, physicians, people who are working with patients. What other groups of researchers might you not automatically think of if you're thinking, “AI for Health, that's computer science, that's medicine”? Who else do you want to see involved in this research? I imagine there's a lot more people who are contributing to this from various departments.
CL: Absolutely. Well, essentially a big one is public health, right, so of course, you know, we are launching our new school of public health, and there's just a lot of social programs and interventions and public health policies. They can be really made a lot more precise and driven by data and driven by AI.
So, you know, we have research where we work on, for example, social welfare and economic intervention strategies for children's health outcomes. And you can predict what policies would be more impactful, would be more effective than others using this data-driven machine learning approaches.
SB: Okay, I like that. So AI, I was thinking about devices and all this cool tech in the hospital, but also for policy, right? You're looking at all the data.
CL: Absolutely. Absolutely. For example, pandemic preparedness, right? So, recall all this debate and confusion about, you know, when should you have the mask mandate? When should you close schools? These are hugely impactful decisions. You want to get it right.
Actually, you know, a lot of research are happening that you can enable these policies to be a lot more data-driven, a lot more precise, based on these AI predictions. So, you want to be able to leverage AI to predict if you close the school today, how that would impact the infection rate, the mortality rate in the community, how that would impact mental health. So, you can have much more informed policymaking in the public health domain.
SB: Okay, amazing. Thank you. That really broadens how I was thinking about this. I want to shift gears a little again to your specific work. So, in addition to all of these things you're doing with building communities and getting more folks involved in AI and health, you're also running your lab here in McKelvey. Tell me more about that. I imagine it's, you know, AI and health related, but what do you specifically focus on in your own research program?
CL: We're having a lot of fun doing AI for health research. So, I mentioned, you know, we are very into wearables, and the combination of wearables and AI, and what we can do with it. Wearable is really in a very interesting place.
For the first time in human history, you know, physicians actually have a sort of readily available tool that they can keep track of patients' conditions after they leave the hospital, in their regular daily lives.
You know, I can't recall how many, say, surgeon friends have told me that they worry about their patients because after major surgery, the patients get discharged, they go back to their normal lives, and they won't see them until, if they're lucky, six months later and sometimes a year later, sometimes even longer. So, a lot of times the conditions worsen, and without the clinicians knowing about it and being able to take measures to prevent and mitigate the problem.
And also importantly, not only these devices are cheap and people are, you know, willing to wear them, which is very, very important. So, in fact, in one year alone, I think it's 2021, 500 million wearables were sold in that one year worldwide. So, that basically means, you know, as I said, for the first time, we can actually get a lot of data, right.
So, but at the same time, as great as these data are – these data are amazing, they are fine-grained. You'll get those measurements every minute. But at the same time, there's too much.
SB: Right, that’s a lot of data.
CL: No physicians and nurses are going to look at these minute-by-minute time series data and say, “Oh, you have depression.” So, that's not how it does.
Basically, this is where AI really comes in. So, this perfect combination of collecting these abundant data in daily lives, that's fine-grained, but also admittedly super noisy. But then you have these powerful AI algorithms that's able to extract reliable health information and insights from these very complicated and imperfect data.
So, this is one area that we're really excited about. For example, we have, in addition to the depression screening project we mentioned a little earlier, we work a lot on surgery. For example, one of the big problems is with high-risk surgeries, such as, for example, pancreatic surgery.
Pancreatic surgery is the only cure for pancreatic cancer, but at the same time, are associated with a very high rate, in fact, as high as 40% of complication rate, after the surgery.
We started working on this when a surgeon came to me and said, you know, look, we really have this problem where every time we have a pancreatic cancer patient, and we have to discuss whether this patient should go for surgery or not. Then it boils down to that question of whether this patient belongs to that 40%, who are going to develop severe complications after the surgery, then they should not have taken the surgery because those complications are going to degrade their life expectancy in fact, and also severely suffer from degradation in their life quality.
But, on the other hand, of course, if you belong to the 60%, you should go for it, and it would cure cancer, right. So, but it's a very difficult decision because the clinicians have very little to go by. It's a tough decision on the clinician, tough decision on the patient, on their families. So, it would be really nice if we could develop a predictive capability for these to be able to predict before surgery whether this patient will have a successful surgery.
So, then we developed this protocol. Basically, the patient would come in and see the surgeon for surgery planning. We would give the patient a Fitbit wristband. The patients would wear the Fitbit wristband for a month. Then, of course, for the clinical study, they would go on with, some of them go on with the surgery. We know the outcome. Using that training data, we train this predictive model. So, for the new patient when they come in, we would be able to, based on their month-long Fitbit data, predict the risk level of this patient.
So, of course, if they are low risk, they go for the surgery, that would be the recommendation of AI. And if they don't, if they're too high, too high a risk, then there are, in our surgery department, they have these prehabilitation programs where they – it’s a nutrition and exercise program – they try to strengthen and improve the condition of the patient before they go for surgery again.
And of course, these are cancer patients. So, delaying their surgery is risky because cancer is developing, so that's why we have to make truly highly reliable predictions. Again, this is where advanced AI become truly important.
SB: Okay. And so with that, you're doing that months-long, you know, you're getting the Fitbit data. I'm thinking about the data of my sleep and my steps and things, that feels very distant from what's going on in my pancreas, for example. So I'm wondering, are there more markers, or are those sort of basic Fitbit markers truly enough for the AI to be able to make those kinds of predictions about the likelihood of complications?
Because those seem very different in seriousness. In terms of like, “Oh, there's cancer happening,” and then like, “Did I get my 10,000 steps today?”
CL: Right. That's a very important question. So intuitively, right, so what surgery does to a person is basically a significant impact on our system. And generally, it boils down to, if it's someone who is in very good condition and very fit, they would be able to sustain that impact and have a successful outcome. Versus someone with, you know, you have high frailty, right, as sometimes put it, people put it in the community, then they were a lot more vulnerable to a major surgery like that. So, in some sense, you are really detecting the frailty of a patient based on this multi-modality data.
And of course, it's never that straightforward. These are complex patterns that the AI is looking for. So, this is why you have to use fairly advanced techniques for it.
SB: Okay.
CL: So, another thing I want to mention is this is also why it's so important to be able to do model interpretation. So, this is something, so in medicine and health in general, people never trust the model if the model cannot explain why I'm making such a serious prediction, such as depression, for example. Fortunately, right now we do have tools where we can actually explain the most important factors that are associated with that particular prediction for this particular individual patient.
This is really interesting because in that depression study, we build these complex deep learning models – it’s called WearNet – that can predict depression, right, based on the Fitbit data. But in the end, we did model interpretation. And we tried to identify what are the most important variables that are associated with the prediction. You know what turns out to be the largest factor?
SB: What?
CL: The total number of steps you take a day.
SB: Oh no, how many should we really be taking? Is it 10,000?
CL: That, we didn't quantify that. But this is really interesting because this is exactly consistent with the mental health literature, which basically said your step count is associated with your risk for depression, which is really satisfying or reassuring in the sense you have this super complex neural network model that ends up discovering the same number one risk factor as all the mental health literature.
For example, the model also discovered that, you know, frequent smoker is a lot more likely to have depression. That's again, it's identical to what had been found in the mental health literature.
So I often joke about it where, you know, you don't want depression, you walk more and you quit smoking, right, which is consistent, but this is also what the deep learning model found.
And of course, deep learning model can do a lot more than traditional statistical studies in health care that found the same concordance, but because it also can mine the complex patterns of these data and make personalized predictions about the individual's risk for depression.
SB: Okay. Yeah, on the one hand, it feels like, you know, you have these super advanced technologies, right, the AI finding stuff that, you know, your doctor's been telling you for years, like it's very bad for you to smoke and you should get outside and have a walk more often, right, like those seem like things that you always hear.
But do you find it sort of reassuring, comforting that the, you know, the advanced techniques do align in that way?
CL: Absolutely, right. So this gives us confidence, right, in how the model works.
SB: How many steps a day do you go for?
CL: Oh, I think my 14,000 steps a day.
SB: Oh my gosh. That's incredible. Okay, well, I need to step up what I'm doing for sure.
CL: And I'm walking around our beautiful campers and beautiful neighborhoods around here.
SB: Okay, okay. Well, that's, I think that's good advice, news we can all use, is that we need to be doing more steps and of course, no smoking. We already have a no smoking campus, though, so I feel like we're on top of that, but more movement. Okay. Love that. Thank you, Chenyang, for that advice.
And I always like to close these conversations by asking for a media recommendation. I'm always looking for something great to read myself or watch. So, I'm curious to hear from you, as an AI expert, what are your favorite media recommendations in books, TV, movies, or wherever of artificial intelligence? And why do you like those?
CL: Yes, I think my recommendation is going to be controversial, but that's a recent Jennifer Lopez sci-fi movie called Atlas.
SB: Atlas, okay.
CL: It's on Netflix. It gets mixed reviews. That's why it's probably, you know, it's controversial. But, it really is a wonderful illustration of sort of human-AI interaction.
So basically, in this movie, there is this robot that J.Lo sits in the robot and they’re accomplishing tasks together. And the robot would have this AI agent having conversation with J.Lo and decide what to do, make recommendations and so on. So it, the conversation, by the way, almost sounds like chat GPT because it's hilarious in that way.
But it really has this deeper issue behind it, which is this very fundamental issue of sort of human-AI interaction. And how does human and AI collaborate and communicate to accomplish tasks together? So interestingly, this actually happens. It's a very, very important issue in medicine. And in health care.
For example, we have AI looking at clinical images, and we have radiologists looking at the same images. They discover different things, and how do they work together in the most effective fashion so that collectively you'll reach the best decisions, and in the most efficient manner? So this is very important questions that you have to ask.
There's also interesting sort of ethics implications in that movie, in the sense of when AI shouldn't listen to human and, you know, those kind of questions.
I'm not saying the movie necessarily made the right recommendations or concordance about these really vital issues about human-AI relationships. But certainly they pose the right questions on a pretty good way.
SB: Yeah. I like that. And I feel like that issue of collaboration is one that is ongoing, right? Like not just sort of handing over control to the machines, but how does this work together in a way that is productive? They're sort of both bringing things to the table. I will count on you to keep me informed as that develops in the health care space as well.
CL: Absolutely. My pleasure.
SB: Thank you so much, Chenyang, for joining us today. This was a delight.
CL: It's my pleasure. Always happy to talk about AI for health.